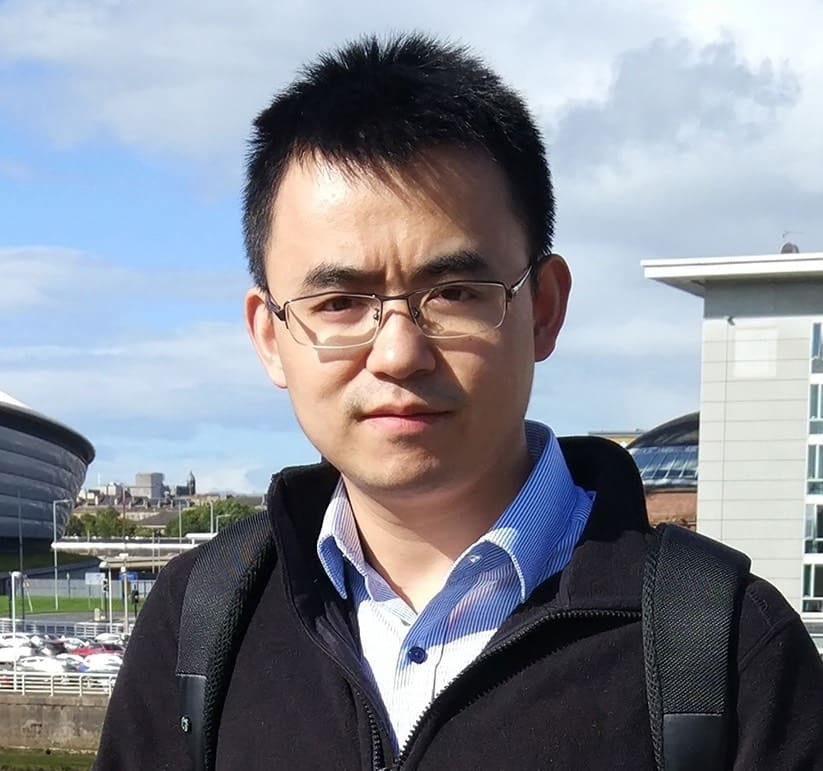
Yang Cao
The Academy’s Digital and Physical Infrastructures team spoke to Academy Awardee Dr Yang Cao about how better data management could enable better system outputs.
Yang explains the importance of data quality and management to enable accessible AI as well as the need to ask the right questions when building and deploying systems.
What are your hopes for the development and deployment of generative AI?
We should start with an understanding of what generative AI is. When we are talking about generative AI, we are largely talking about chat bots or, in more technical terms, large language models. These are deep learning models that are trained on internet-scale datasets, but the way they work is actually quite intuitive and simple. From the user's perspective, we give a prompt—a sentence or even a question—then the model generates a string of words as a response. The answers are almost what you would expect from a real human, but really, the AI system is just recursively predicting what word sequences are most likely to constitute a complete response.
At the moment, most of the discussion on generative AI is around these types of chatbots like ChatGPT. However, there are wider forms of AI, including generative AI, that are of value. Image video analysis, for instance, can create value in sectors like healthcare and autonomous transport in a way that text generation cannot – and I am eager to see what the possibilities are when the attention of the big models is redirected.
Do you have any fears about the progress and implementation of generative AI?
I am worried about the huge capacity to produce misinformation that language models have. For example, coders and software engineers use a very popular website called Stack Overflow for coding questions. Contributions from ChatGPT have been specifically banned from the site because of how likely they are to contain false or untrustworthy information.
Chatbots are inclined to speak in an authoritative way, even when they are talking nonsense – so it can be quite easy to receive and spread misinformation they have generated without realising it. Generative AI has made misinformation very easy to spread at little cost, which is particularly concerning in the contexts of political propaganda and fraud. I fear that unless we can put accountability in place around what is generated by AI products, we will only see more and more misinformation.
I am also a bit worried about the current wave of optimism about generative AI. Current conversation has the potential to portray an overly optimistic external view of the capability of generative AI. Unless we have a realistic view of generative AI and what it can and cannot achieve, there is a risk that when it does not meet our expectations it will damage the image of AI, machine learning, or data science more broadly.
At this juncture, what questions do we need to be asking ourselves to enable the safe and impactful use of AI?
This is really important. The first question we should ask is whether a task is suitable for AI. Is generative AI or large language models or even machine learning suitable for the task at hand? Not everything can positively benefit from AI.
In a recent workshop at the University of Edinburgh, we learned about an interesting case along these lines. It involved a lawsuit in the US where the lawyers used ChatGPT to generate a seemingly well-written statement of findings referencing previous legal cases that supported their position. During fact-checking, however, it was identified that the cases cited had been made up by ChatGPT.
This is an important case for the improper use of generative AI in a high-stakes application. Now, it does not mean that generative AI cannot be used in law, but it is a prime example of why we should first ask whether this is feasible. What is the risk assessment? What if the machine learning model does not work correctly as expected? Can we accept the outcome and consequences?
I fear that unless we can put accountability in place around what is generated by AI products, we will only see more and more misinformation.
What are the respective roles of researchers, those deploying the technologies, regulators, and policy makers in enabling safe use of generative AI?
Each stakeholder plays an important role, however their influence on the sector is currently unequal.
Researchers, especially those from academia, have not been able to participate fully because of budget and lack of access to large data sets to train big models. Researchers need to participate to enable the safe use of AI as big tech companies have a disproportionately large power in controlling the development and deployment of AI.
Academic research on how the big models could be used more fairly and responsibly is difficult to carry out because we simply don’t have the resources. But researchers can still make an impact particularly in terms of regulation, accountability, and how data is managed, collected, protected and secured.
Regulators and policymakers are in a position to create policies for developers on setting accountability for how models are developed and used. Policymakers are also in a unique position to oversee how models access our data and how public data should be governed.
Policymakers are also in a unique position to oversee how models access our data and how public data should be governed.
What is not being talked about in the ongoing media discussion around generative AI?
Everyone seems quite optimistic, but currently even the big models are limited in their capabilities., We mostly focus on the best-case scenarios when we talk about generative AI. We emphasise how they can behave like a human in conversation, but we need to pay more attention to their limitations.
For example, students can use chatbots to help with written exams, but it has also been reported that the responses generated by these models are sometimes of poor quality. If we are going to make best use of those models for education, we should be clear on what they can and cannot do.
I would also like to see more research on data management, because the capabilities of these big language models are limited by the data they are using. At the moment, they are consuming internet-scale databases, meaning that if we want more value from these models we need more data – and better data. So, while traditional research on data quality usually focuses on database and data science applications, I think there are opportunities for more systematic research on data quality for machine learning as well.
Related content
Data and AI
The Academy has undertaken a wide range of projects on the role of data and artificial intelligence (AI) in shaping the…