RAEng / Leverhulme Trust Research Fellowships 2024
Dr Perelli is developing a new framework for automated quantitative material decomposition in X-ray Computed Tomography scans using photon counting detectors (PCD-XCT) and generative deep learning, with the aim of addressing the rise in CT exams and associated X-ray dose risks in the UK. Traditional methods require time-consuming and costly manual image segmentation by radiologists. PCD-XCT offers high-resolution, sensitive imaging at lower doses but struggles with noise at low doses. The project combines PCD-XCT physics with unsupervised deep learning to directly decompose tissues from scan data, bypassing manual segmentation. This end-to-end system aims to reduce costs and enhance robustness in low-dose tissue estimation, significantly impacting radiological quantitative imaging accuracy.
Dr Perelli aims to develop a new framework for automated quantitative material decomposition using photon counting detectors x-ray computed tomography PCD-XCT and generative deep learning for accurate diagnosis.
From a UK national perspective, the number of CT examinations has increased dramatically from two to six million between 2014 and 2020 with the consequent growth in X-ray dose, which poses safety concerns. Current clinical procedures involve CT image reconstruction followed by manual segmentation, performed by radiologists to identify crucial features, and decompose the image into separate tissue images such as bone, fat, which is extremely time consuming and costly.
PCD-XCT is a radically new technology that can provide the required spatial resolution and material discrimination sensitivity at much lower radiation doses. However, the material decomposition of PCD-XCT is an ill-conditioned problem that significantly magnifies the noise and limits its clinical value at low radiation doses. Deep neural networks have been applied to a variety of imaging applications; however, PCD-XCT presents difficult problems because of the high-dimensional data and the non-linearity of the physics model.
The project aims to develop an automated pipeline able to decompose material images from PCT low-dose measurements without the need of manual segmentation. The framework is based on the concept of combining the knowledge of the physical model of PCD-XCT with unsupervised deep learning diffusion models to decompose tissues directly from the scanner’s data.
The proposed end-to-end deep-learning system will segment tissue images using PCD-XCT at reduced costs and improve the robustness of quantitative tissue estimation for pre-clinical low-dose PCD-XCT. The outcomes will have an immediate impact in radiology where it is of outmost importance to assess the accuracy of quantitative imaging under low-dose conditions.
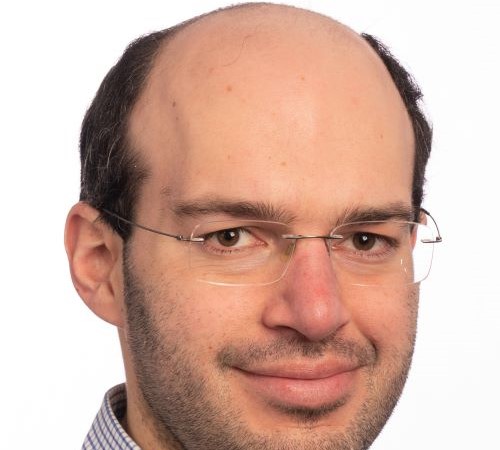
Related content
View all programmesSupport for research
The Academy runs a number of grants to support excellent researchers carry out engineering activities and to enable clo…
Research Chairs and Senior Research Fellowships
This scheme aims to strengthen the links between industry and academia, supporting academics in UK universities to unde…